13 May 2025
HKU CDS Public Lecture: How Over-Parameterization Slows Down Gradient Descent by Prof. Simon Du
We investigate how over-parameterization impacts the convergence behaviors of gradient descent through two examples. In the context of learning a single ReLU neuron, we prove that the convergence rate shifts from $exp(−T)$ in the exact-parameterization scenario to an exponentially slower $1/T^3$ rate in the over-parameterized setting.
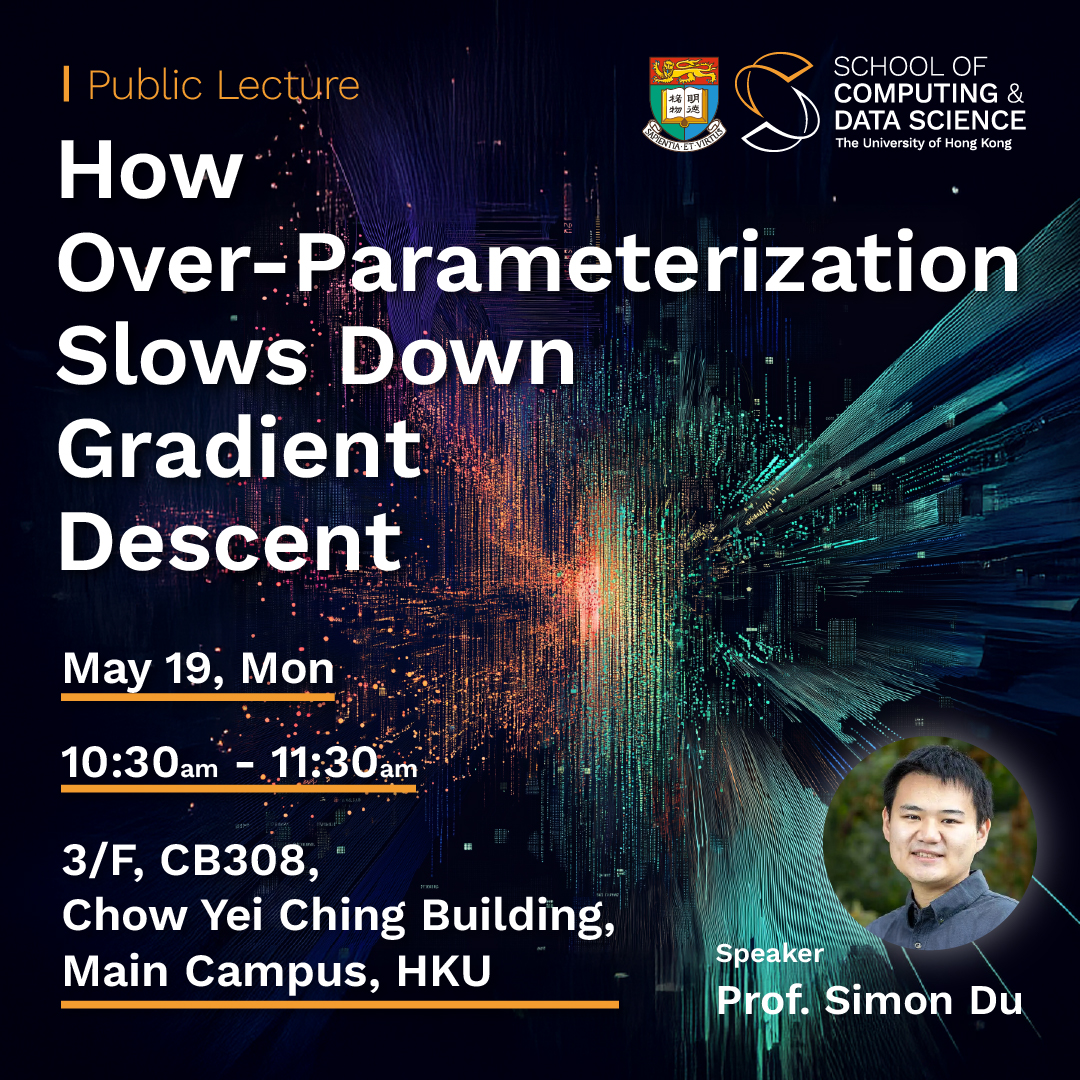
Speaker:
Prof. Simon Du, Paul G. Allen School of Computer Science & Engineering, University of Washington
Date:
19 May, 2025 (Monday)
Time:
10:30 am – 11:30 am
Venue:
3/F, CB308, Chow Yei Ching Building, Main Campus, HKU
Abstract:
We investigate how over-parameterization impacts the convergence behaviors of gradient descent through two examples. In the context of learning a single ReLU neuron, we prove that the convergence rate shifts from $exp(−T)$ in the exact-parameterization scenario to an exponentially slower $1/T^3$ rate in the over-parameterized setting. In the canonical matrix sensing problem, specifically for symmetric matrix sensing with symmetric parametrization, the convergence rate transitions from $exp(−T)$ in the exact-parameterization case to $1/T^2$ in the over-parameterized case. Interestingly, employing an asymmetric parameterization restores the $exp(−T)$ rate, though this rate also depends on the initialization scaling. Lastly, we demonstrate that incorporating an additional step within a single gradient descent iteration can achieve a convergence rate independent of the initialization scaling.
Biography:
Simon S. Du is an assistant professor in the Paul G. Allen School of Computer Science & Engineering at the University of Washington. His research interests are broadly in machine learning, such as deep learning, representation learning, and reinforcement learning. Prior to starting as faculty, he was a postdoc at the Institute for Advanced Study of Princeton. He completed his Ph.D. in Machine Learning at Carnegie Mellon University. Simon’s research has been recognized by a Sloan Research Fellowship, an IEEE AI’s 10 to Watch Fellowship, a Schmidt Sciences AI2050 Early Career Fellow, a Samsung AI Researcher of the Year Award, an Intel Rising Star Faculty Award, an NSF CAREER award, a Distinguished Dissertation Award honorable mention from CMU, among others.
All are welcome to attend.